Explore the foundations of data science with Statistics and Hypothesis Testing. Master essential concepts to analyze and interpret data effectively. Elevate your skills in hypothesis testing for informed decision-making. Dive into this comprehensive guide for data-driven insights.
Explore essential concepts, methods, and techniques to analyze data effectively and make informed decisions. Perfect for both beginners and seasoned professionals looking to enhance their data analysis skills. Learn how to analyze data, make informed decisions, and draw meaningful conclusions using statistical methods tailored to your needs.
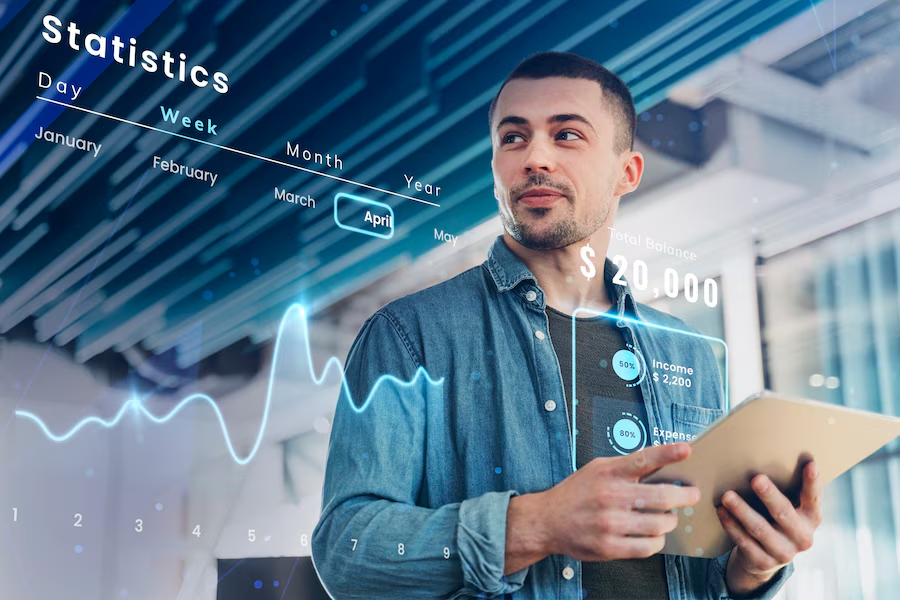
Understanding Statistics in Data Science:
Statistics serves as the backbone of data science, offering a systematic approach to understand, interpret, and draw conclusions from data. At its core, statistics involves the collection, organization, analysis, interpretation, and presentation of data. In the realm of data science, statistical techniques are employed to explore relationships, identify patterns, and make predictions.
1. Descriptive Statistics: Descriptive statistics provide a summary of the main features of a dataset. Measures such as mean, median, mode, variance, and standard deviation offer insights into the central tendency and spread of the data. Visualization tools like histograms, box plots, and scatter plots aid in visually representing the characteristics of the data.
2. Inferential Statistics: Inferential statistics enable data scientists to make inferences and predictions about a population based on a sample of data. Techniques such as hypothesis testing, regression analysis, and confidence intervals are utilized to draw conclusions and make predictions with a certain level of confidence.
Hypothesis Testing: Unravelling Truths from Data:
Hypothesis testing is a fundamental concept in statistics that allows data scientists to evaluate assumptions, test hypotheses, and draw conclusions about populations based on sample data. The process typically involves the following steps:
1. Formulating Hypotheses: The first step in hypothesis testing is to formulate a null hypothesis (H0) and an alternative hypothesis (H1). The null hypothesis represents the status quo or a statement of no effect, while the alternative hypothesis contradicts the null hypothesis and asserts a specific effect or relationship.
2. Choosing a Significance Level: The significance level (α) is the probability of rejecting the null hypothesis when it is actually true. Commonly used significance levels include 0.05 and 0.01, representing a 5% and 1% chance of Type I error, respectively.
3. Collecting Data and Calculating Test Statistics: Data is collected and analyzed to calculate a test statistic, which serves as the basis for evaluating the hypotheses. The choice of test statistic depends on the type of data and the hypothesis being tested.
4. Making a Decision: Using the test statistic and the chosen significance level, a decision is made whether to reject the null hypothesis or fail to reject it. This decision is based on comparing the test statistic to a critical value or calculating the p-value, which represents the probability of observing the data if the null hypothesis is true.
5. Interpreting Results: Finally, the results of the hypothesis test are interpreted in the context of the problem being studied. If the null hypothesis is rejected, it indicates evidence in favor of the alternative hypothesis. Conversely, if the null hypothesis is not rejected, it suggests insufficient evidence to support the alternative hypothesis.
Applications of Statistics and Hypothesis Testing in Data Science:
The applications of statistics and hypothesis testing in data science are vast and diverse, spanning various industries and domains:
1. Business Analytics: In business analytics, statistical techniques are used to analyze market trends, forecast sales, optimize marketing campaigns, and evaluate business performance.
2. Healthcare: In healthcare, statistics plays a crucial role in clinical trials, epidemiological studies, disease surveillance, and healthcare outcomes research.
3. Finance: In finance, statistical models are employed for risk management, portfolio optimization, asset pricing, and quantitative trading strategies.
4. Manufacturing: In manufacturing, statistics is utilized for quality control, process improvement, defect detection, and supply chain optimization.
Conclusion Statistics And Hypothesis Testing:
Statistics and hypothesis testing form the bedrock of data science, providing the tools and techniques necessary to extract actionable insights from data. By leveraging these methodologies, data scientists can unlock the hidden potential of data, drive informed decision-making, and fuel innovation across various domains. As the field of data science continues to evolve, a solid understanding of statistics and hypothesis testing remains essential for success in unlocking the true value of data.
statistics and hypothesis testing serve as the backbone of data science, enabling practitioners to derive meaningful insights and make informed decisions from complex datasets. By mastering statistical concepts and methodologies, data scientists can effectively analyze data, identify patterns, and draw reliable conclusions. Hypothesis testing provides a rigorous framework for validating assumptions and drawing inferences about population parameters.